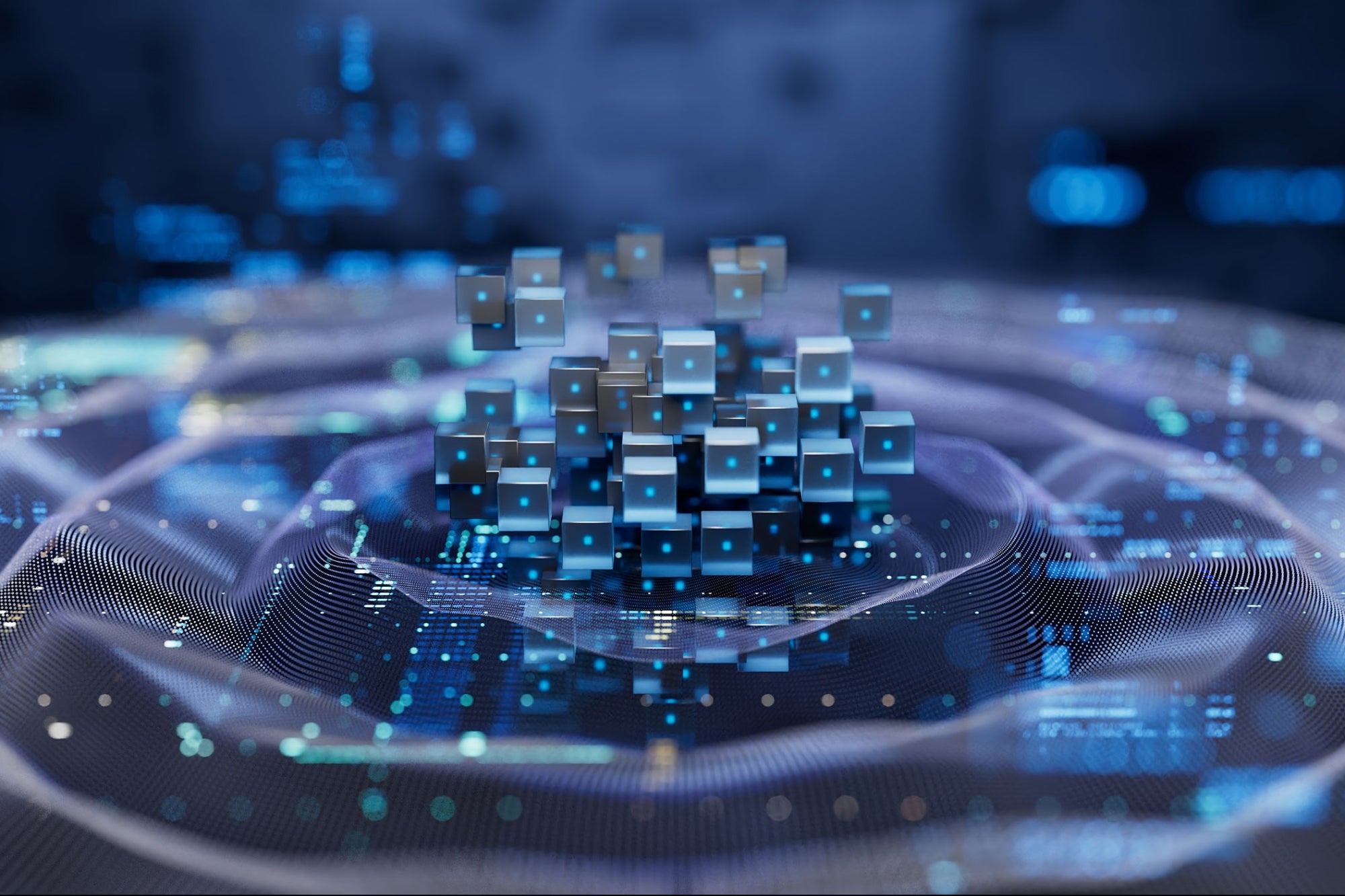
Opinions expressed by Entrepreneur contributors are their own.
In today's digital world, businesses are realizing the limitations of their old data systems. With AI and advanced analytics, businesses are forced to rethink data management. Modern data architectures enable scalability, better accessibility, real-time insights and efficient resource optimization.
Modern data architecture is a no-brainer for new businesses. It is revolutionary in scaling and maintaining adaptability in your organization's data needs. Integration and accessibility for your data are at an all-time high while providing insights in real-time.
Data is protected in new ways, is more secure, and is more cost-efficient. Modern data architecture revolutionizes automation and creates highly diverse and quality data. Therefore, there are numerous benefits to modernizing your data architecture.
What is modern data architecture?
Modern data architecture is how data is structured and stored in organizations. It involves all major data processes: collection, storage, access, usage, management and protection. Past versions of data architecture were primarily focused on getting through the day-to-day work. Today, data architecture is modernized and more about deriving insights and getting more out of our data. Modern data architecture is cloud-based and focused on analytics.
Modern data architecture is flexible but ensures that the data is still manageable. Organizations can seamlessly scale their data volumes following their growth and progress. Automation of high-quality data is at the forefront of modern data architecture, with security and flexibility embedded throughout.
This leads to the top seven reasons for adopting modern data architecture.
1. Scalability and flexibility
Modern data architecture is designed for new and revolutionary business needs. It encompasses cloud computing, AI and big data and thus needs to be able to store, process and analyze data at large scales. This scalability means that larger amounts of data need to be handled in the same way that smaller amounts are handled currently.
With a large influx of data, horizontal and vertical scaling are necessary. Horizontal scaling allows data to be spread across multiple additional servers, while vertical scaling involves upgrading the existing servers. Data partitioning can help organize this data, while sharding can help distribute the data across numerous servers. With such large scaling, data replication can help maintain data integrity in the event of failure.
Related: 3 Reasons Why You Need Data To Scale Your Company
2. Enhanced data integration and accessibility
At this point, data needs to be integrated across multiple platforms and sources. Big data do not drive decision-making, meaning data integration is changing. Some of the major methods include extract, transform, load (ETL), extract, load, transform (ELT), change data capture (CDC), application programming interface (API), federated data mesh and event-driven architecture.
These are used for extracting data from several different sources, and transforming it and then loading it into a database or loading it and then transforming it. CDC is for real-time data changes, while APIs are for data communication between an endpoint and source. Federated data meshes create customized data products, while event-driven architecture notices events within data for real-time response. These all give greater accuracy to the data and, beyond that, greater accessibility.
Related: Why Vertical Integration Allows Leaders to Actually Control Their Data
3. Real-time analytics and insights
Data is not only centered around daily use now, it needs to be analyzed and tracked in real-time. Insights can be drawn more perceptively on real-time data. This gives businesses the ability to make better-informed decisions and allows them to operate on higher efficiencies.
This is revolutionary as modern data architecture can ingest data from tens of thousands of sources simultaneously. It can validate, clean up, normalize, transform and enrich this data to provide driven, guided and insightful responses. This is remarkable and gives businesses a serious edge in the modern age.
4. Improved data governance and security
Just as the need for data has evolved exponentially, so has the governance and security behind it. Everyone is involved in this process. Decentralized control is imperative for widespread data spreading while fostering accountability across all stakeholders.
Data lineage helps to keep track of all processes and procedures in a timely look-through. This shared responsibility and sharing of the data itself also help foster accountability since everyone is important and involved. The zero trust model helps protect private and public applications and extends past traffic verification, where traditional network architecture stops.
Related: Your Data Is Useless If You Don't Have a Management Strategy
5. Cost efficiency and resource optimization
Since all data in modern data architecture is primarily stored in the cloud, there are large capabilities for cost savings and operational efficiency. It does not even need to be entirely cloud-based for it to classify as modern, it can be multi-cloud or hybrid as well.
Choosing data solutions will allow you to obtain new data in a much less painful and quicker, cost-effective way. In modern data architectures, you typically only pay for what you use as well as all your data processing is significantly streamlined, offloading a lot of computational costs. Decoupled resources are particularly useful for helping scalability and allows for several queries at once on data.
6. Automation
With high data usage requirements, automation is important. It can help reduce errors and draw insights and feedback from all users and sources to maintain oversight over the entire data structure. This creates a more trustable model and can also allow for automated updates, which will help to release security patches efficiently.
Orchestration and metadata can make automation possible and faster, and artificial intelligence (AI) and machine learning (ML) can be used for data discovery, processing and enrichment, consumption, auto-scaling and validation.
7. Diverse and quality data
Current times require structured and unstructured data, which allows for transformative use. This is pivotal because it yields higher-quality and more usable data. The users of data are also more diverse, which calls for diversification of the data. Everyone is analyzing, collaborating and innovating. Modern data architecture optimizes tools for data cleaning, enrichment and governance to yield high-quality data. To achieve diverse data, it utilizes collection, storage, analysis and utilization techniques.